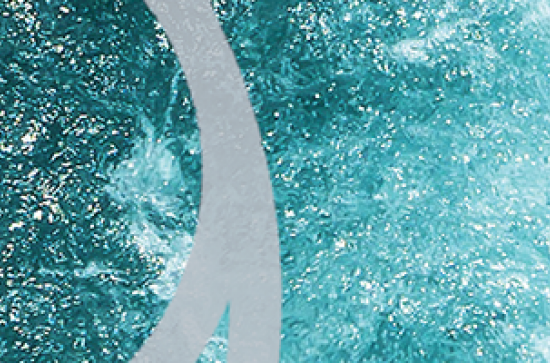
Virtual participation: Zoom details available here
Speaker: Dr Vasudevan Nedumpozhimana, Trinity College Dublin
Title: "Topic aware probing: From sentence length prediction to idiom identification how reliant are neural language models on topic?"
Abstract: Transformer-based neural language models achieve state-of-the-art performance on various natural language processing tasks. However, an open question is the extent to which these models rely on word-order/syntactic or word co-occurrence/topic-based information when processing natural language. This work contributes to this debate by addressing the question of whether these models primarily use topic as a signal, by exploring the relationship between Transformer-based models’ (BERT and RoBERTa’s) performance on a range of probing tasks in English, from simple lexical tasks such as sentence length prediction to complex semantic tasks such as idiom token identification, and the sensitivity of these tasks to the topic information. To this end, we propose a novel probing method which we call topic-aware probing. Our initial results indicate that Transformer-based models encode both topic and non-topic information in their intermediate layers, but also that the facility of these models to distinguish idiomatic usage is primarily based on their ability to identify and encode topic. Furthermore, our analysis of these models’ performance on other standard probing tasks suggests that tasks that are relatively insensitive to the topic information are also tasks that are relatively difficult for these models.
Biography: Vasudevan Nedumpozhimana is a research fellow at the ADAPT Centre, Trinity College Dublin. Vasudevan completed his PhD at the Indian Institute of Technology Bombay, India and was a Marie Skłodowska-Curie (EDGE) research fellow at Technological University Dublin. His research interests include Natural Language Processing, Distributed Semantics, Compositionality, and Language Models.
Reference: Nedumpozhimana V, Kelleher JD. “Topic aware probing: From sentence length prediction to idiom identification how reliant are neural language models on topic?” Natural Language Processing. doi:10.1017/nlp.2024.43