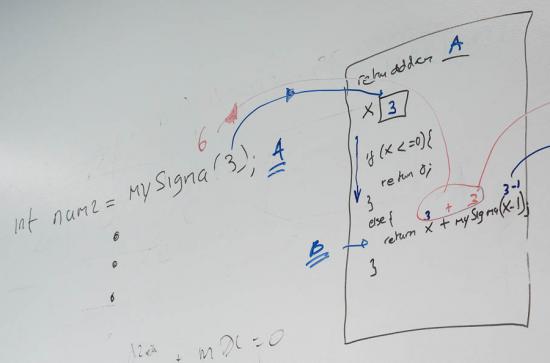
Speaker: Professor Nial Friel, School of Mathematics and Statistics, University College Dublin
Title: "Informed sub-sampling MCMC: Approximate Bayesian inference for large datasets"
Abstract: This talk introduces a framework for speeding up Bayesian inference for large datasets. We design a Markov chain whose transition kernel uses an (unknown) fraction of (fixed size) of the available data that is randomly refreshed throughout the algorithm. Inspired by the Approximate Bayesian Computation (ABC) literature, the subsampling process is guided bythe fidelity to the observed data, as measured by summary statistics.
The resulting algorithm, Informed Sub-Sampling MCMC (ISS-MCMC), is a generic and flexible approach which, contrary to existing scalable methodologies, preserves the simplicity of the Metropolis-Hastings algorithm. Even though exactness is lost, i.e. the chain distribution approximates the posterior, we study and quantify theoretically this bias and show on a diverse set of examples that it yields excellent performances when the computational budget is limited.
This is joint work with Florian Maire (Montreal) and Pierre Alquier (INSAE, Paris).
Reference: Maire, F., Friel, N. & Alquier, P. Informed sub-sampling MCMC: Approximate Bayesian Inference for Large Datasets. /Statistics and Computing/ (2018).