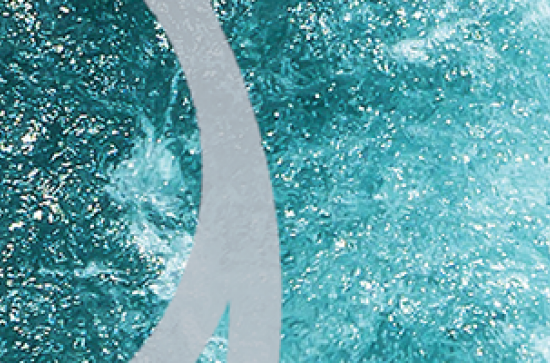
Speaker: Dr Chih-Hong Cheng, Fraunhofer IKS
Title: "When neural networks meet dependability"
Abstract:
Neural networks (NN) have been introduced in safety-critical applications from autonomous driving to train inspection. I argue that to close the demo-to-product gap, we need scientifically rooted engineering methods that can efficiently improve the quality of NN. In particular, I consider a structural approach (via GSN) to argue the quality of neural networks with NN-specific dependability metrics. A systematic analysis considering the quality of data collection, training, testing, and operation allows us to identify many unsolved research questions: (1) How to create a weaker form of data completeness while admitting the combinatorial explosion of scenarios? (2) How to overcome the specification problem for formal verification, where input as images can be hard to characterize? (3) How to justify that a decision made by the network is supported by prior similarities in the training data? Some of my conducted prior work will be concisely mentioned with a statement on their limitations.
Bio:
Chih-Hong Cheng is currently a researcher at Fraunhofer IKS, acting as the head of the research department on safety assurance for AI. His research interests include software engineering, formal methods, and AI/ML for trustworthy autonomy. He had extensive collaborations with leading companies in domains including industrial automation, railway, and automotive. Apart from his daily duties, he also serves as an international expert (subteam lead) for the ISO 8800 publicly available specification in safety and AI for automotive. Chih-Hong obtained his doctoral degree from TU Munich.
Virtual Participation: Join Zoom
Meeting ID: 880 1818 5733
Passcode: 279596