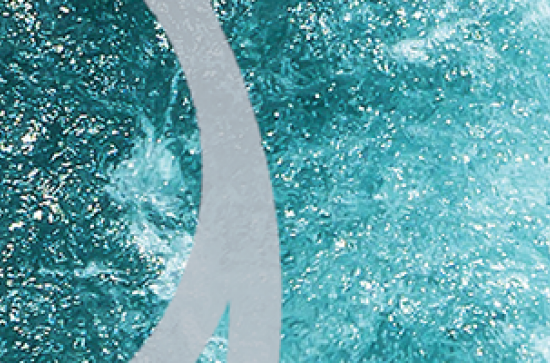
https://us02web.zoom.us/j/88018185733?pwd=OEcxb2ZSYUwzV2hWTE9wcE1yTWRUQT09
Passcode: 279596
In-person: Hamilton Institute Seminar room (317), 3rd Floor Eolas Building, North Campus, Maynooth University
Speaker: Dr Mimi Zhang, Trinity College Dublin
Title: "Reinforced EM Algorithm through Clever Initialization for Clustering with Gaussian Mixture Models"
Abstract: Model-based clustering methods are ubiquitous in applications. Typically, such methods employ the Expectation Maximization (EM) algorithm for parameter estimation. However, EM has the notorious yet unsolved problem that the initialization input significantly impacts the algorithm output. Recent exemplar-based likelihood methods provide an alternative approach to initialize cluster means. By assuming that the clusters are dense enough such that there is always a data point very close to the real cluster center, then the cluster means can be approximated by such “exemplars” in the dataset, and the mean-initialization problem transforms to exemplar selection. However, existing exemplar-based initialization approaches all treat every data point as a candidate exemplar and therefore must impose strict assumptions on the mixture components to make the computational complexity a↵ordable. With the motivation to tackle the initialization problem for model-based clustering, we here develop a powerful EM-type algorithm. Our contributions are four-fold: (1) we apply a fast peak-finding technique to generate an inclusive set of exemplars, the size of which is much smaller than original data size; (2) our regularized objective function is convex and well justified in the context of mixture modelling; (3) our Gaussian mixture components are free to take any form of covariance; (4) our algorithm is fast and high performing. We present theoretical guarantees for the quality of the recovered exemplars and experimental results to verify that our algorithm works well in practice.