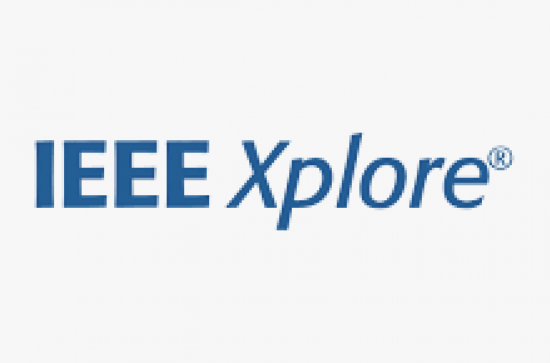
Abstract:
There are many difficulties in managing and detecting preterm pregnancies, especially in the early stages. Analyzing electrohysterogram data, which show the electrical activity of uterine muscles, is a promising non-invasive method for classifying term and preterm pregnancies. However, the effectiveness of machine learning classifiers is hampered by the inherent class imbalance in datasets. In order to overcome this constraint, this study uses a Generative Adversarial Network to produce high-quality synthetic EHG data while maintaining the statistical properties of actual signals. By successfully reducing class imbalance, the enhanced dataset makes it possible for machine learning models to be trained with resilience. Real and synthetic features did not differ significantly, according to statistical validation using a t-test (p > 0.05). With this method, the Random Forest classifier obtained a ROC-AUC of 0.99 and a 10-fold cross-validation accuracy of 98%. The suggested GAN-based method’s simplicity and efficacy demonstrate its promise for resolving class imbalance and developing reliable classification systems for diagnostic applications.
Date of Publication: 23 April 2025
Publisher: IEEE
Read the new publication in Q1 journal.
Authors
Muhammad Omar Cheema
Department of Biomedical Engineering, Air University, Islamabad, Pakistan
Zia Mohy Ud Din
Department of Biomedical Engineering, Air University, Islamabad, Pakistan
Azhar Imran
Department of Creative Technologies, Faculty of Computing & AI, Air University, Islamabad, Pakistan
Fawad Salam Khan
Department of Creative Technologies, Faculty of Computing & AI, Air University, Islamabad, Pakistan
Mahmood Basil A. Al-Rawi
Department of Optometry, College of Applied Medical Sciences, King Saud University, Riyadh, Saudi Arabia
Mohammed El-Meligy
College of Computer Science and Informatics, Amman Arab University, Amman, Jordan
Jahan Zeb Gul
Department of Electronic Engineering, Maynooth University, MAYNOOTH, Ireland