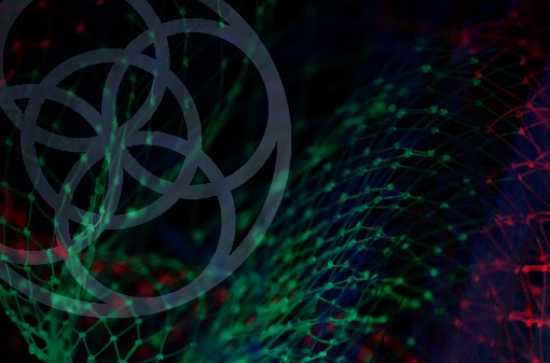
Accurate understanding of mobile network traffic at city scale is increasingly important for precision network engineering, demand-aware allocation of compute resources, and security. Gaining such knowledge is however difficult, because it requires large-scale measurement collection using dedicated equipment, substantial storage capabilities, and non-trivial post-processing.
In this talk I will first make the case for harnessing the power of deep learning to obtain highly-accurate real-time mobile traffic analytics that can fuel the management of 5G networks. I will then show how machine learning can be used in a principled way to detect network threats in their infancy and how to build such detection systems with a view to deployment at the edge.